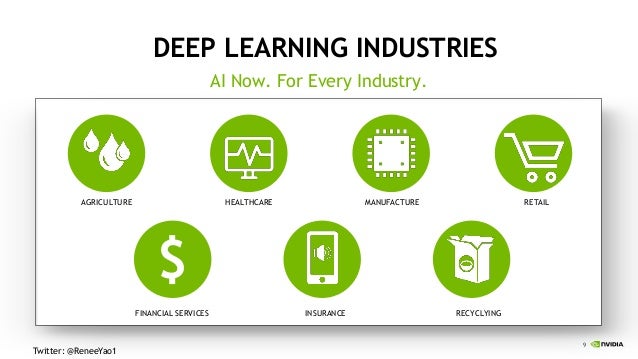
How does it work
To make the long story short, understanding deep learning algorithms amounts to understand two aspects: architecture and back-propagation.
The architecture of a neural network involves multiple neurons, interacting between themselves, in successive layers. These are as many parameters (number of layers, type of interactions, weights on each cells), that the machine will “learn” by confronting this architecture to real world data.
Here comes the second element: back-propagation. The idea is very simple: every time a new input enters the network, the output gives an error (from a loss function). Then this error is back-propagated so that each neuron is attributed a contribution to the error. The contribution to the error is used to optimize weights by gradient descent, in order to ultimately minimize the loss function.
Anyway the real magic is that in deep learning, everything is optimized simultaneously.
These are the high level principles on the method, the purpose is not to dig into the technical details. Instead one can focus on the key elements driving success in deep learning.
Practically speaking, what is needed in order to make it working?
- Large volume of data: because there are hundreds of thousands of parameters to calibrate, the number of observations must be way bigger!
- A certain type of input data, where the added-value is proven. This is typically unstructured data: image, text, and audio. The intuition here is that complex and deep neural networks bring a high added value when the variables used are rather complex to infer. This is typically the case for images, text files, and audio files.
- Deep learning has less proved significant breakthrough in other applications with structured data, as compared to baseline machine learning algorithms.
Let’s try to illustrate with two standard examples: image and text.
One of the major classes of deep learning architecture used for image processing is Convolutional Neural Networks. They typically identify and focus on various parts of an image, and then assemble them in order to achieve classification of images. These methods will learn the contours, the forms, on the left, on the right, the contrast level, etc. This is the high added value part. Then decision made by the machine relies on relatively simple rules.
Anytime that the data contains a historical or temporal dimension, the deep learning architectures used are called Recurrent Neural Networks, and among them one of the most popular is the LSTM standing for Long-Short Term Memory.
They have loops in themselves so that they can take into account the past (short term and long term). Information becomes persistent. The most famous example is the one of inferring a word in a sentence. The memory effect is needed in order to infer the very last word of the following sentence: “I have been leaving in Germany for 5 years … I am fluent in German”. In this example a standard model could learn that a word following “fluent in” will be the name of a language. In order to predict that this language is German, the model needs to incorporate a memory effect: “Germany” appears earlier in the sentence.
LSTM can also be seen as the equivalent of time series’ econometrical models in the deep learning field. It is strongly believed that there is still large innovation opportunities in this kind of approaches, both in finance (where many time series are at stake, obviously), and also in the insurance industry, where time series and space-time series are becoming omnipresent (we will come back on this point in the next paragraph).
ليست هناك تعليقات:
إرسال تعليق